Understanding Machine Learning: A Comprehensive Guide
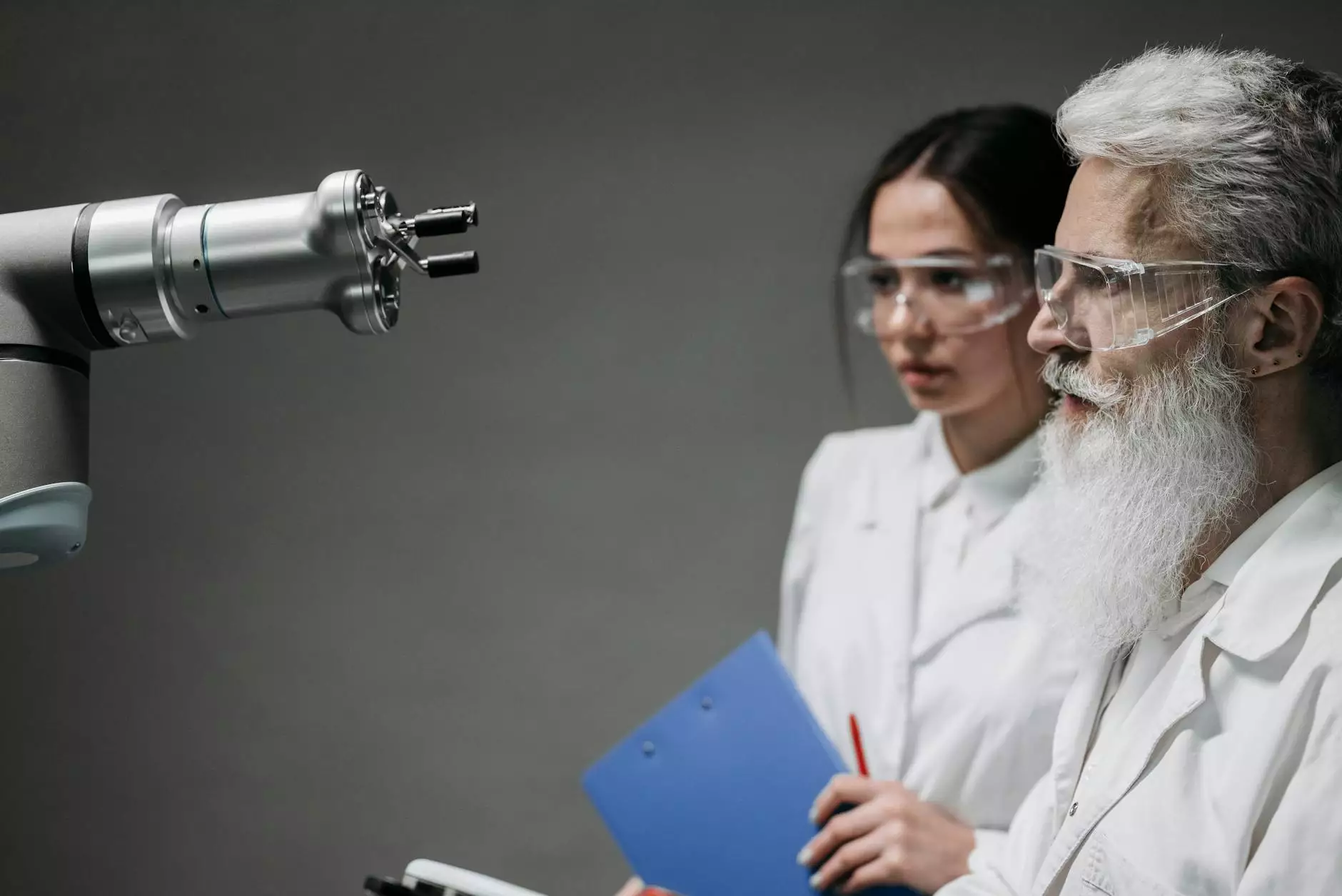
In today's rapidly advancing technological landscape, machine learning has emerged as a pivotal force driving innovation and efficiency across various industries. The aim of this article is to provide an in-depth understanding of machine learning, covering what it is, how it works, its applications, the benefits it offers, and the future of this transformative technological domain. We will delve into key concepts, methodologies, and case studies that illustrate the power of machine learning in real-world scenarios.
What is Machine Learning?
At its core, machine learning is a subset of artificial intelligence (AI) that focuses on developing algorithms that enable computers to learn from and make predictions based on data. Rather than being explicitly programmed to perform a task, these algorithms analyze patterns in data and improve their performance over time through experience. This ability to learn from data with minimal human intervention is what sets machine learning apart from traditional programming methods.
The Evolution of Machine Learning
The concept of machine learning dates back to the mid-20th century. Over the decades, it has evolved from basic statistical models to complex neural networks capable of performing tasks that were once thought to be exclusive to human intelligence. This evolution has been driven by several factors:
- Increased Data Availability: The proliferation of big data has provided vast amounts of information for training machine learning models.
- Advancements in Computing Power: The rise of powerful GPUs and cloud computing has made it feasible to process large datasets efficiently.
- Innovative Algorithms: Continuous research and development in algorithms have led to more efficient and effective machine learning techniques.
How Does Machine Learning Work?
Understanding how machine learning works involves grasping a few key components:
1. Data Collection
The foundation of any machine learning model is data. This data can come from various sources, including databases, online resources, and sensors. The quality of the data is crucial, as it directly impacts the model's performance.
2. Data Preprocessing
Before training a model, the data must be cleaned and prepared. This process may involve handling missing values, eliminating noise, and performing feature scaling to ensure consistency across the dataset.
3. Choosing a Model
There are numerous machine learning algorithms to choose from, categorized into:
- Supervised Learning: Algorithms are trained on labeled data, learning to associate inputs with outputs. Common examples include regression and classification tasks.
- Unsupervised Learning: Algorithms work with unlabeled data to identify patterns and groupings. Clustering and dimensionality reduction are common uses.
- Reinforcement Learning: Models learn through trial and error, receiving rewards or penalties based on their actions.
4. Training and Testing
After choosing a model, it is trained on a portion of the dataset (training set) and validated on another portion (test set). This process helps to evaluate the model's accuracy and generalizability.
5. Deployment and Monitoring
Once the model meets performance expectations, it can be deployed in real-world applications. Continuous monitoring and updating may be required to maintain performance as new data becomes available.
Applications of Machine Learning
Machine learning has a diverse range of applications across various sectors. Some notable examples include:
1. Healthcare
In healthcare, machine learning algorithms analyze medical records, predict patient outcomes, and assist in diagnosing diseases. These systems can identify patterns that may not be apparent to human practitioners, leading to improved patient care.
2. Finance
Financial institutions utilize machine learning for fraud detection, credit scoring, and algorithmic trading. By analyzing transaction data, these systems can flag suspicious activities in real-time, enhancing security.
3. Retail
In the retail sector, businesses employ machine learning for customer segmentation, inventory management, and personalized marketing strategies. Algorithms can predict consumer behavior, allowing retailers to optimize their offerings and improve customer satisfaction.
4. Manufacturing
Machine learning enhances production processes through predictive maintenance. By analyzing equipment data, companies can foresee potential failures before they occur, reducing downtime and saving costs.
5. Transportation
Self-driving cars are a notable application of machine learning, where algorithms process data from sensors and cameras to navigate and make real-time decisions on the road.
Benefits of Machine Learning
The implementation of machine learning brings several advantages:
1. Increased Efficiency
Machine learning algorithms can automate repetitive tasks, freeing up human resources for more complex activities. This efficiency boost can lead to significant cost savings.
2. Enhanced Decision Making
By filtering through vast amounts of data quickly, machine learning models can provide decision-makers with actionable insights, enhancing the overall decision-making process.
3. Personalization
Machine learning allows for personalized experiences by analyzing user data and preferences. This capability is particularly valuable in marketing, where tailored content can significantly improve engagement rates.
4. Predictive Analytics
The ability to predict future trends based on historical data is one of the most powerful aspects of machine learning. Organizations can leverage this to anticipate market shifts and customer needs.
5. 24/7 Availability
Unlike human employees, machine learning systems can operate continuously, allowing for round-the-clock data processing and real-time responses.
The Future of Machine Learning
The future of machine learning is poised for transformative growth, with several trends expected to shape its development:
- Automated Machine Learning (AutoML): As demand for machine learning grows, simplified platforms are emerging, allowing non-experts to build and deploy models.
- Explainable AI: With increasing emphasis on transparency, future developments will focus on models that can explain their decisions, making them more trustworthy.
- Integration with IoT: The Internet of Things (IoT) will propel machine learning, where algorithms will analyze data from connected devices to enhance automated processes.
Conclusion
Machine learning is not merely a buzzword but a profound technological advancement that has already begun reshaping our world. Its ability to learn from data and improve over time is empowering businesses to operate more efficiently, make informed decisions, and adapt to changing circumstances. As we look ahead, the continued evolution of machine learning promises even greater possibilities, reinforcing its role as a cornerstone of innovation in the digital age. Embracing machine learning isn't just an option—it's a necessity for businesses aiming to thrive in a competitive environment.
For more information on machine learning strategies tailored to your business, explore machinelearningconsulting.net.
article about machine learning